Table of Contents
Introduction to Discrete Sampling Techniques
Discrete sampling techniques are quite crucial in a variety of fields ranging from statistics to data science and even machine learning. These methods are intended for discrete data, which has individual and varying values, unlike continuous data, which are capable of any value between fixed or given. Due to their concentration on separated values, these methods allow for correct measurement, interpreting and predicting the unique aspects of the phenomenon when results are measurable and existential.
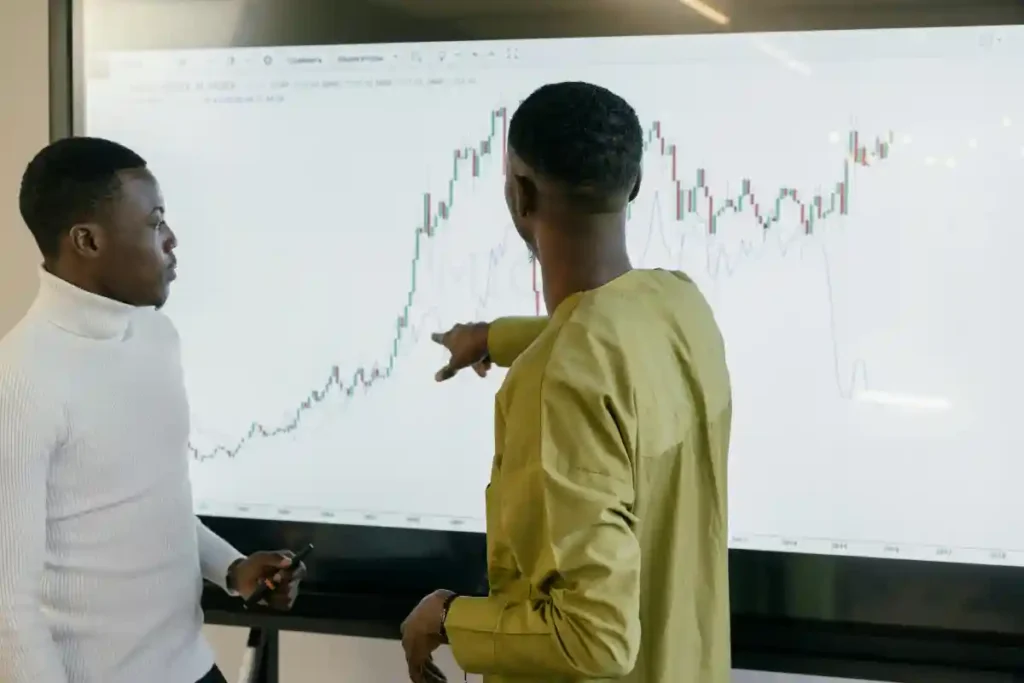
TCDModelSamplingDiscrete: Precision in Discrete Data Analysis
Discrete sampling is used in statistics to help determine the values of several categorical variables, including survey responses and experimental results. This makes it possible for the researcher to make inferences about the state of population parameters and to test hypotheses as well. Data science employs discrete sampling for operations such as pattern analysis, categorization, and feature extraction, especially where data is organized. On the other hand, discrete sampling techniques used in machine learning enable several algorithms that need labelled data for training and testing to improve and achieve better results.
TCDModelSamplingDiscrete can be considered a significant development of discrete sampling solutions. It provides a sketchy and less complicated way to deal with these discrete datasets effectively. This model uses complex techniques in sampling procedures, hence increasing the validity and reliability of research and data findings. As a result, its implementation into data science pipelines has the potential to improve the accuracy of prediction models and statistical tests.
While in continuous sampling the data points are allowed to have any quantitative value, discrete sampling is more selective in the sense that it is more appropriate for data that are quantized and categorical. By its very nature, as designed for discrete data, TCDModelSamplingDiscrete is an essential asset to practising professionals working in different disciplines. Hence, it reduces the complexity because it increases the precision and reliability of the sampling process, which in turn enhances advanced research and real-life application.
Key Concepts and Terminology
Sampling Frame Basics
This paper starts with the concepts and definitions that are core to discrete sampling by laying down important assumptions about this area of study. The term’ sampling frame’, therefore, refers to the list or the database from which the sample is going to be taken. It is important, especially in view of making sure that those selected into the sample are true representations of the population. The “sample size” refers to the number of units taken from the sampling frame to be used as a sample; the issue is crucial as it determines the reliability of the results to be obtained.
Random Sampling Basics
Random sampling’ is an approach whereby each element in the population has a probable chance of being chosen, thereby reducing biases. This method belongs to probability theory, which focuses on the ‘probability distribution’, or the function that gives the probability of an event. Knowledge of these distributions is useful in forecasting and assessing patterns in a given set of data.
Basics of Random Sampling
Depending on the nature of the research and its goals, many distinct sampling techniques are used. Among them is the ‘simple random sampling’ method, in which each unit is selected solely based on chance. This is an easy process that could cover a wide area, but it may, at times, be time-consuming or restricted for large populations.
Order-Based Sampling
Systematic sampling’ also simplifies unit selection by choosing them at a regular interval within the sampling frame, e.g., every 10th unit. Calculating the arithmetic mean for the desired population is a simpler and easier technique for applying a sample mean. However, this technique also presupposes that the population is ordered randomly, so it may not be accurate most of the time.
Subgroup Sampling Technique
Stratified sampling’ and ‘stratified random sampling ‘entails partitioning the whole sampling population into separate groups or ‘strata’ and then taking a random sample from each of these. In this method, subpopulations are included based on important characteristics yielding accurate results than those of simple random samples.
Cluster Sampling Explained
Cluster sampling’ makes this a lot easier by grouping the population in clusters, often by geographical, family, or other natural formation. Then, groups are randomly chosen, and all the units of each group are interviewed. This is particularly useful when working with large and dispersed populations.
Through such concepts, it becomes easy to apply tcdmodelsamplingdiscrete to gain effectiveness in data analysis from various resources.
Applications and Case Studies
Discrete sampling techniques are important, particularly in various disciplines, and TCDModelSamplingDiscrete has been demonstrated to be useful in a broad range of applications. In this section, a detailed elaboration of the testing of the tool TCDModelSamplingDiscrete for any given speciality or discipline and particular case-study scenarios will be provided.
It is important when it comes to survey designs in the domain of statistical analysis within the social sciences since it works with limited populations and the process is called discrete sampling. For instance, a study that sought to identify the voting behaviour pattern applied a discrete sampling technique, TCDModelSamplingDiscrete, to generate sub-samples from the total population. Due to the proper division of the population, the results produced by the researchers are accurate and efficient for prediction models to make effective strategic plans for political campaigns.
Most machine learning-based processes involve the need to partition data comprehensively for model training and evaluation. An example is a top financial technology firm that applied TCDModelSamplingDiscrete in order to develop a better fraud detection mechanism. There were issues in dealing with datasets which were skewed in the sense that the number of fraudulent transactions was significantly low compared to normal transactions. By adopting the use of discrete sampling techniques, the firm could be able to develop well-balanced datasets that would enhance the training of the algorithm to identify and eliminate fraudulent activities while at the same time ensuring that the accuracy levels of the algorithm were improved and the false positives were reduced.
Discrete sampling methods are widely used in manufacturing processes, particularly in quality control. One of the large automobile companies successfully applied TCDModelSamplingDiscrete to track the quality of engines. In this way, the company could quarantine large batches of production and take small samples of parts for thorough examination. It also brought about quality control, eliminating shoddily made products while reducing wastage and expenses. Such information was useful in identifying areas of ongoing improvement for the company’s operations.
These case studies prove that TCDModelSamplingDiscrete and other discrete samples are powerful tools for addressing various issues in various fields. Whether trying to increase the probability of attaining accurate survey results, designing a better machine learning structure, or keeping a tight QC standard, discrete sampling is an influential means for getting the right end product.
Conclusion
Most of the time, throughout this extensive guideline, we have expounded on the details and specifications of the discrete sampling approaches with special regard to TCDModelSamplingDiscrete. Comparing these fundamentals with the methodologies and applications associated with them, it is apparent that these two techniques have huge potential in many spheres. Given the fact that TCDModelSamplingDiscrete can handle and analyze discrete data sets, it shows how the model explains efficacious solutions to several computational challenges.
Therefore, the exploration of TCDModelSamplingDiscrete and discrete sampling techniques provides a vast universe of opportunities and prospects for development. Thus, by adopting these methods, researchers and practitioners can open brand new avenues in their disciplines respectively. Therefore, we recommend that readers refer to these techniques and read more about how one can harness these methods to the full to achieve growth and success in any given endeavour.