Today, with globalization starting to dominate all sectors of human life, translation is more important than ever. Today, MT systems are indeed more and more essential to bridge the language gap; one of the most critical features of these systems is to give good translations. To solve this crisis, the latest Qwen2 machine translation model with Qwen2的BLEU指标 is one of the main measures used to assess the quality of the translation.
In this blog, we will discuss how Qwen2 uses BLEU, the benefits of using such an approach, and the effectiveness of the strategy in ensuring accuracy in translations.
Table of Contents
Understanding BLEU Metrics
The overall quality of the translation in an MT System is measured mostly by the BLEU score, which is commonly used in the technique. Having been described in detail since the early 2000s at the latest, Qwen2的BLEU指标 provides nothing but a strictly numerical indication of how much the MT reproduces one or several reference translations. The better the quality of the translated text, the higher the BLEU scores, which generally range from 0 to 1.
The premise of Qwen2的BLEU指标 is based on evaluating n-gram precision, which is two consecutive terms of n from the translated content. For instance, when an MT system uses phrases similar to the reference translation, the matching phrases are added to the Qwen2的BLEU指标 score. Moreover, BLEU penalizes short translations, extending the belief that the translation length is important.
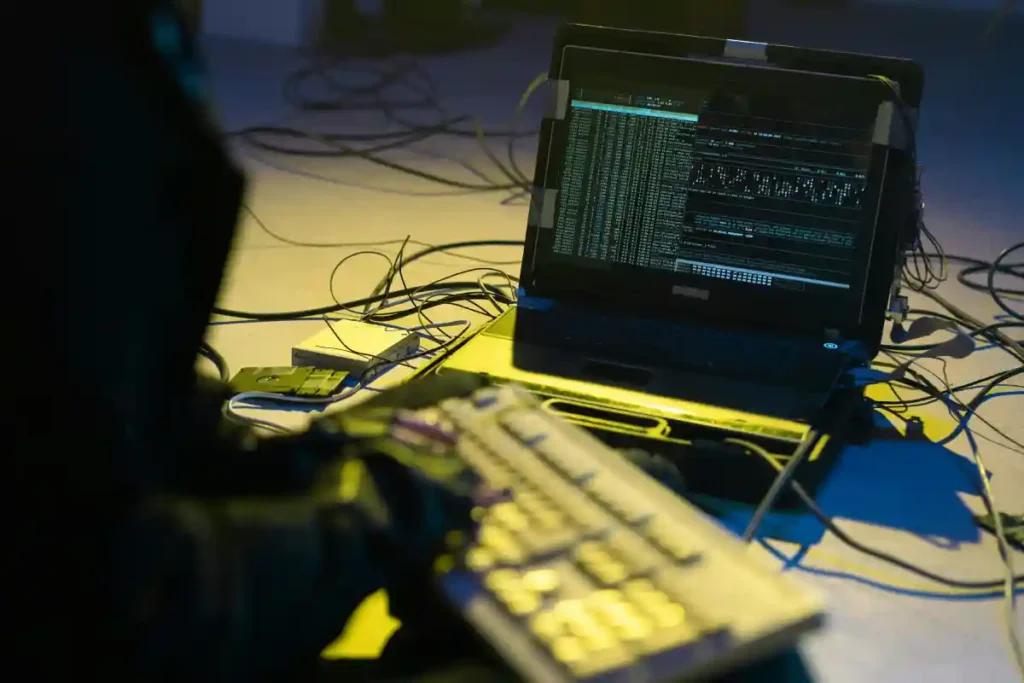
How Qwen2 Utilizes BLEU Metrics
Qwen2’s BLEU metrics in several innovative ways to optimize translation accuracy:
Real-Time Evaluation
Qwen2的BLEU指标 has impressive performance metrics; among the points, it is possible to mention using BLEU scores in real-time feedback. While translations are being produced, Qwen2的BLEU指标 dynamically produces the BLEU score so users can see their likeness to high-quality reference texts. This immediate feedback system can allow users to evaluate the quality of the translations in terms of their utility for a given purpose in writing or for business use.
Training Optimization
Qwen2的BLEU指标 scores as one of its central types of training. In training, the model computes BLEU scores and then diagnoses the corresponding strengths and weaknesses of the translations that it produces. Qwen2 adapts its performance to these assessments as it modifies its algorithms accordingly. Such an iterative learning process enhances the descriptive adequacy of the model, thus providing better translation results.
Comparative Output Analysis
Another feature in Qwen2的BLEU指标 is that when translating, the program can provide several outputs for one input. Using the BLEU scores for these different outputs, the actual output from the model can be sorted based on the degrees of their quality. This feature allows users to select the most appropriate translation since they can easily choose one from many options.
User-Centric Customization
The second strength of using Qwen2 to address BLEU metrics is that program users can input their reference translations. This custom-made feature enables the model to assess relative to context, fields or individual tastes. Because Qwen2的BLEU指标 integrates much more closely into user requirements than Babelfish, the latter can offer translations closer to the target group.
Advantages of Using BLEU Metrics in Qwen2
The integration of BLEU metrics into Qwen2 offers several key advantages:
Standardization of Evaluation Research
While other metrics aren’t available for BLEU comparison, the assessment of Qwen2’s performance is more accurate because it has a steady comparison system. This kind of standardization helps provide clear scores of what the users should expect from the model as the performance reports become more transparent.
Emphasis on Precision and Content Preservation
The scoring based on n-gram matches deployed in BLEU prioritizes preserving content and structures from the source text. This feature is crucial for translation because it helps to maintain the message of the required focus and convey it in the target language.
Measurement in terms of performance indicators
BLEU scores are measurable so that users can determine the difference between the two particular models between or after the training sessions. By evaluating these scores, users can determine the efficiency of diverse translation approaches and methods and settle for the best solution that meets specific translation requirements.
Support for Multiple Languages
Qwen2的BLEU指标 can be used on a large number of language pairs, so it would be a great asset to Qwen2 if it included translation in multiple languages. This capability enables the model to adapt to various users, making it more desirable and feasible.
Impact on Translation Accuracy
The incorporation of BLEU metrics into Qwen2 has had a profound impact on the quality of translations produced by the model:
Enhanced Accuracy
Through the recurrent modifications of its outputs in response to BLEU assessments, Qwen2 has exhibited enhanced capability in translation efficiencies. Such an iterative cycle enables the model to correct mistakes and generate outputs even closer to the intended meaning of the text.
Reduction in Errors
Using BLEU scoring, the program is also capable of detecting common flaws in translations so that Qwen2 can avoid making such mistakes continuously. Such a feedback loop minimizes the chances of repeated blunders, giving more accurate and credible inter lingual translations.
increased User Satisfaction
Users have praised the option to select reference translations and noticed improved satisfaction with Qwen2 outputs. Therefore, the model can adjust to certain contexts and nee, ds, and the expected user translation quality is enhanced.
How to Effectively Benchmark a Model against Others
It is preferable to compare with other translation models, and by doing this, BLEU proves to be more useful than the method used above. This benchmarking helps compare and contrast features between Qwen2 and other systems and challenges researchers to develop more efficient machine translation methods.
Conclusion
Integrating BLEU metrics into the Qwen2 machine translation model significantly advances the quest for accurate and efficient translations. By employing BLEU for real-time evaluation, training optimization, and comparative output analysis, Qwen2的BLEU指标 continually enhances its translation quality. The advantages of standardized assessment, emphasis on precision, and adaptability to user needs make BLEU an invaluable tool in this context. As Qwen2的BLEU指标 continues to evolve, its commitment to leveraging robust evaluation methods like BLEU is set to redefine the standards of machine translation, making it an essential tool in today’s interconnected world.